Projects
Ongoing
Normative modules: Architecture for cooperative LLM-based generative agents.
Generative agents, which implement behaviors using a large language model (LLM) to interpret and evaluate an environment, has demonstrated the capacity to solve complex tasks across many social and technological domains. However, when these agents interact with other agents and humans in presence of social structures such as existing norms, fostering cooperation between them is a fundamental challenge. In this paper, we develop the framework of a 'Normative Module': an architecture designed to enhance cooperation by enabling agents to recognize and adapt to the normative infrastructure of a given environment. We focus on the equilibrium selection aspect of the cooperation problem and inform our agent design based on the existence of classification institutions that implement correlated equilibrium to provide effective resolution of the equilibrium selection problem. Specifically, the normative module enables agents to learn through peer interactions which of multiple candidate institutions in the environment, does a group treat as authoritative. By enabling normative competence in this sense, agents gain ability to coordinate their sanctioning behaviour; coordinated sanctioning behaviour in turn shapes primary behaviour within a social environment, leading to higher average welfare. We design a new environment that supports institutions and evaluate the proposed framework based on two key criteria derived from agent interactions with peers and institutions: (i) the agent's ability to disregard non-authoritative institutions and (ii) the agent's ability to identify authoritative institutions among several options. We show that these capabilities allow the agent to achieve more stable cooperative outcomes compared to baseline agents without the normative module, paving the way for research in a new avenue of designing environments and agents that account for normative infrastructure.
Completed
Informal institutions and polarization in online social systems
Although there is mounting empirical evidence for the increase in affective polarization, few mechanistic models can explain its emergence at the population level. The question of how such a phenomenon can emerge from divergent opinions of a population on an ideological issue is still an open issue. In this paper, we establish that human normativity, that is, individual expression of normative opinions based on beliefs about the population, can lead to population-level polarization when ideological institutions distort beliefs in accordance with their objective of moving expressed opinion to one extreme. Using a game-theoretic model, we establish that individuals with more extreme opinions will have more extreme rhetoric and higher misperceptions about their outgroup members. Our model also shows that when social recommendation systems mediate institutional signals, we can observe the formation of different institutional communities, each with its unique community structure and characteristics. Using the model, we identify practical strategies platforms can implement, such as reducing exposure to signals from ideological institutions and a tailored approach to content moderation, both of which can rectify the affective polarization problem within its purview.
A generalized cognitive hierarchy model for dynamic games
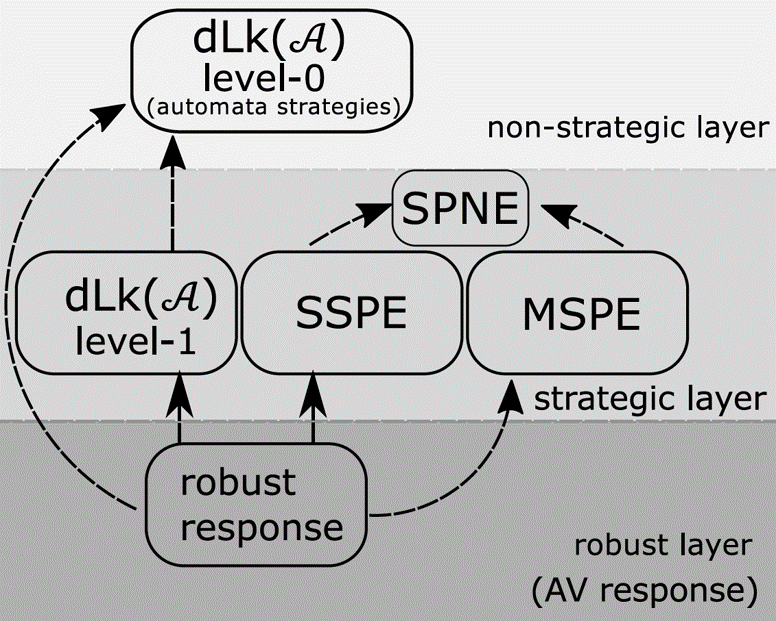
While there has been an increasing focus on the use of game theoretic models for autonomous driving, empirical evidence shows that there are still open questions around dealing with the challenges of common knowledge assumptions as well as modeling bounded rationality. To address some of these practical challenges, we develop a framework of generalized dynamic cognitive hierarchy for both modelling naturalistic human driving behavior as well as behavior planning for autonomous vehicles (AV). This framework is built upon a rich model of level-0 behavior through the use of automata strategies, an interpretable notion of bounded rationality through safety and maneuver satisficing, and a robust response for planning. Based on evaluation on two large naturalistic datasets as well as simulation of critical traffic scenarios, we show that i) automata strategies are well suited for level-0 behavior in a dynamic level-k framework, and ii) the proposed robust response to a heterogeneous population of strategic and non-strategic reasoners can be an effective approach for game theoretic planning in AV
Safety Validation of strategic planners
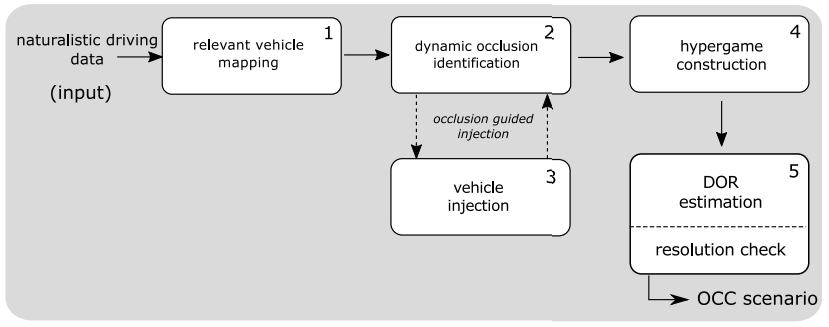
A particular challenge for both autonomous and human driving is dealing with risk associated with dynamic occlusion, i.e., occlusion caused by other vehicles in traffic. Based on the theory of hypergames, we develop a novel multiagent dynamic occlusion risk (DOR) measure for assessing situational risk in dynamic occlusion scenarios. Furthermore, we present a white-box, scenario-based, accelerated safety validation framework for assessing safety of strategic planners in AV. Based on evaluation over a large naturalistic database, our proposed validation method achieves a 4000% speedup compared to direct validation on naturalistic data, a more diverse coverage, and ability to generalize beyond the dataset and generate commonly observed dynamic occlusion crashes in traffic in an automated manner.
Waterloo Multi-Agent Traffic Dataset
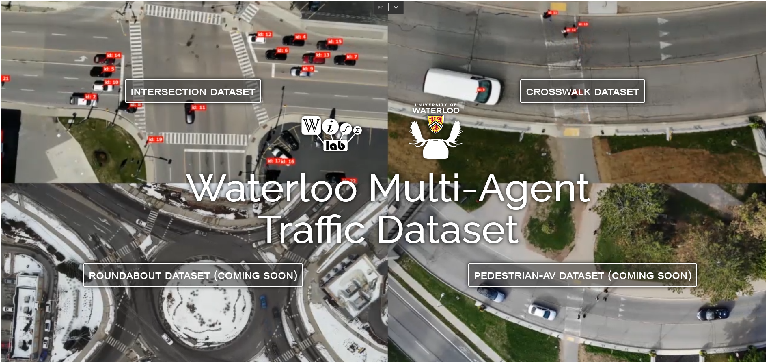
A multi-agent dataset of traffic interactions covering various scenarios, such as, busy intersections, crosswalk interaction with pedestrians, roundabouts, and interaction between pedetrians and AV. The dataset is under active development, and the intersection and crosswalk dataset is available here
Taxonomy of strategic and non-strategic interactions
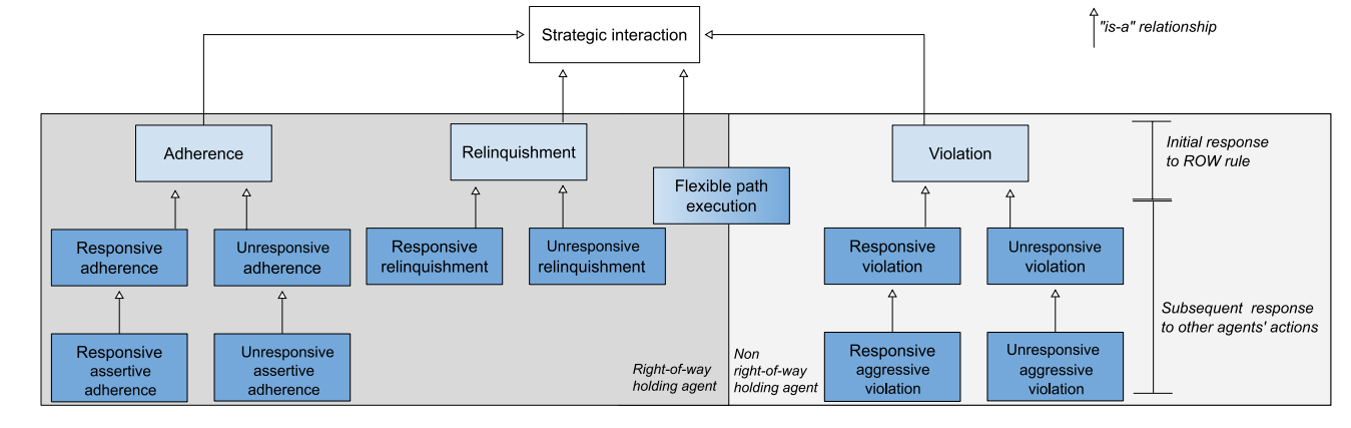
In order to enable autonomous vehicles (AV) to navigate busy traffic situations, in recent years there has been a focus on game-theoretic models for strategic behavior planning in AVs. However, a lack of common taxonomy impedes a broader understanding of the strategies the models generate as well as the development of safety specification to identity what strategies are safe for an AV to execute. Based on common patterns of interaction in traffic conflicts, we develop a taxonomy for strategic interactions along the dimensions of agents' initial response to right-of-way rules and subsequent response to other agents' behavior. Furthermore, we demonstrate a process of automatic mapping of strategies generated by a strategic planner to the categories in the taxonomy, and based on vehicle-vehicle and vehicle-pedestrian interaction simulation, we evaluate two popular solution concepts used in strategic planning in AVs, QLk and Subgame perfect epsilon-Nash Equilibrium, with respect to those categories.